Why Survey Researchers Don't Want "Other" Responses, But Include them Anyway
How and why to answer confusing or badly-written survey questions without choosing "Other"
Have you ever:
Given feedback on a product?
Taken a poll, or your country’s National Census?
Been evaluated for a mental illness?
Participated in a research study?
If so, you’ve taken a survey.
Which means you’ve probably had thoughts like these:
“What does this question mean? Why did they word it like that?”
“My answer doesn’t fit any of the response choices given. Help!”
Especially on mental health questionnaires about frequency or severity: “My answer is somewhere between choices B and C. Which one best fits what I mean?”
For polls: “Answer A fits my opinion, but the whole question is framed in a philosophical or political way I disagree with.”
For demographic questions: “It’s not their business.”
For psychological surveys: “A in some situations and B in other situations, depending on C— but how do I express that?”
“How do I say, ‘I have no idea?’”
If you get frustrated enough, you might stop taking the survey and avoid similar ones in the future.
In situations like these, you’ve probably looked for an “Other” button and its stalwart companion, the write-in box.
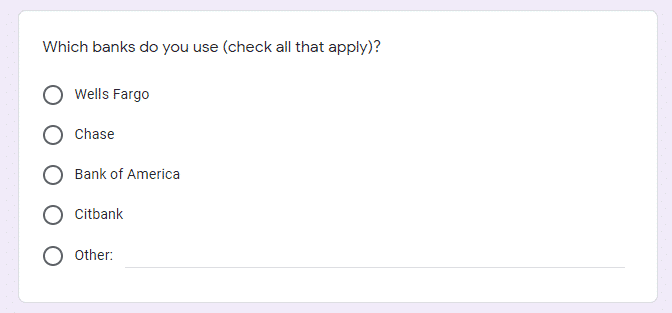
If you’re anything like me, you probably appreciate having these options, and get annoyed when they’re absent. These help you get across your perspective in 2 ways:
The “Other” option lets you convey that none of the given response choices given fits.
The write-in line allow you to explain why they don’t fit and provide your actual response. For example, maybe you use 5th/3rd bank.
Your voice will be heard—if only by an undergraduate research assistant.
So, you might be surprised to learn that researchers want as few “Other” responses as possible.
I’ll explain why, from a researcher’s point of view.
Next time you face an ambiguous survey question, you can better decide how (not) to respond. At the least, you’ll understand why it was written that way.
The Tradeoff Between Inclusion and Information
Imagine you’re creating a survey. You’re writing demographic questions — age, socioeconomic status (SES), etc. Your research team has no questions about demographics. However, you’re obligated to record them anyway, for 2 reasons:
To measure the equitability of opportunities to participate in research,
So researchers who do have demographic questions can analyze your data.
For you, it would be easiest to write a few simple answer choices that represent most survey takers and are easy to code. (More about coding shortly).
However, categories like race/ethnicity, gender, and sexuality are complicated and fraught, requiring more numerous, specific answer choices.
People choose labels that reflect many inner experiences and social-political groupings. “Preferred” words change frequently. Moreover, people passionately disagree about whether it’s acceptable to use certain labels (for example, “queer” as an umbrella term for people with minority underrepresented genders and sexualities). If you choose such a term, it will offend some participants.
So, how much of this complexity can you capture, in as few response choices as possible, while causing minimal offense?
People vary so much that the number of identities you could potentially define is immense —ending only when each “group” contains only 1 person. So, where do you choose to stop dividing?
That’s where “Other” options and write-in boxes enter the picture. These choices ensure that the researchers record every participant’s input, regardless of whether it fits the categories they’re using.
Unfortunately, “Other” responses are hard to analyze quantitatively.
Why “Other” Responses are Hard to Quantitatively Analyze
Statistical analysis compares groups, not individuals.
Suppose you want to know whether people feel less severely depressed after taking a certain amount of Antidepressant X for a certain amount of time. You would divide participants into a “Before Antidepressant X Treatment” and an “After Antidepressant X Treatment” group. If you want to know if Antidepressant X reduces depression more than a placebo does, you would compare a group that receives Antidepressant X to a group that receives the placebo.
The process that follows — null hypothesis testing — confuses people because, instead of showing that something affects something else, you show that it does not have no effect. You don’t show that people are feeling better after taking Antidepressant X; you show that they are not not feeling unchanged or worse. You don’t show that Antidepressant X works better than placebo; you show it doesn’t work the same or worse. (I believe statistical hypothesis testing confuses people partly because they have difficulty understanding double negatives).
Don’t worry about the details. The point is, to include a participant’s data in a statistical analysis, you have to assign them to a group. You can then compare these groups. This process is called “coding” the data.
People who answer a survey question divide into one group per response choice.
So, for the survey question below, “Would you like to enroll in paid services to get products earlier?”, participants fall into 3 groups: those who said “Yes,” those who said “No,” and those who said “Maybe later.” That lets you compare the people who gave each response.
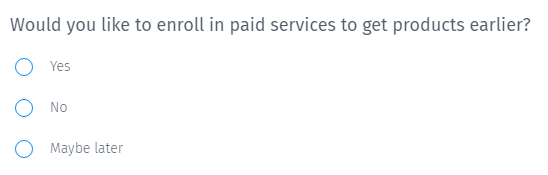
In the example question above, we know what each of these choices (Yes, No, Maybe later) mean, so we also know how to interpret group differences.
So, what happens when a participant says, “Other?”
More specifically, suppose you find a statistically significant group difference between people who say “Other” and people who say, for example, “Yes.” What does that mean?
It depends what “Other” means.
Were they unsure how to interpret the response choices?
Was their real answer in-between 2 response choices? Did they not know which to pick?
Did they mean “sometimes 1 response choice and sometimes another?”
Did they have no opinion?
Did they choose not to answer?
Did they outright disagree with the way you framed the question?
Did each participant mean something different?
You could deal with “Other” responses in different ways.
You could break down the “Other” category into sub-categories for each possible motivation. There are 2 potential problems with this approach:
Not enough information. Participants may not all have explained their reasons for choosing “Other” in the write-in box or survey margins.
Not enough statistical power. The subcategories may include so few people that even if there is a real group difference, any analyses using them will lack the statistical power to detect it.
Personally, I would analyze “Other” as one category, without interpreting the participants’ intent. Ideally, someone would also do a qualitative analysis of the write-in responses…but that’s a whole other study.
Whatever option you choose, the more people give an “Other” response, the more information is lost.
You end up with a tradeoff.
The more “Other” responses your survey question gets, the more people’s perspectives are recorded (the more inclusive the survey), but the fewer you can analyze (the less informative it is).
So, researchers try to write their questions in such a way that as few people as possible select the “Other” option.
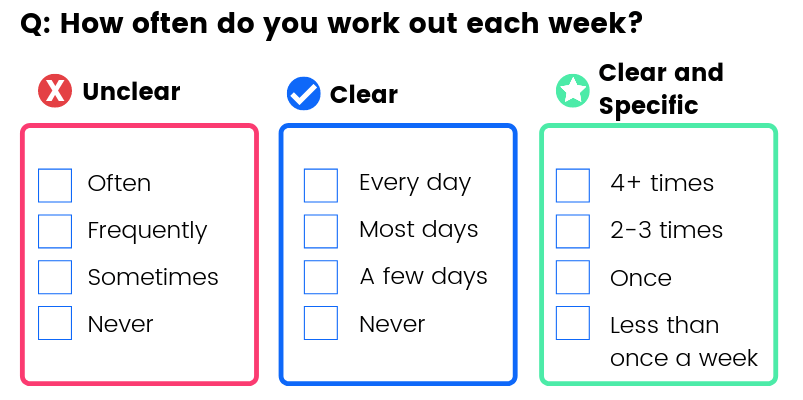
The challenge of writing survey questions is making them flexible enough to cover as many people as possible, yet not so broad and open-ended that they confuse people. As you’ve probably noticed, not everyone gets it right.
How Should You Answer A Badly-Written Survey Question?
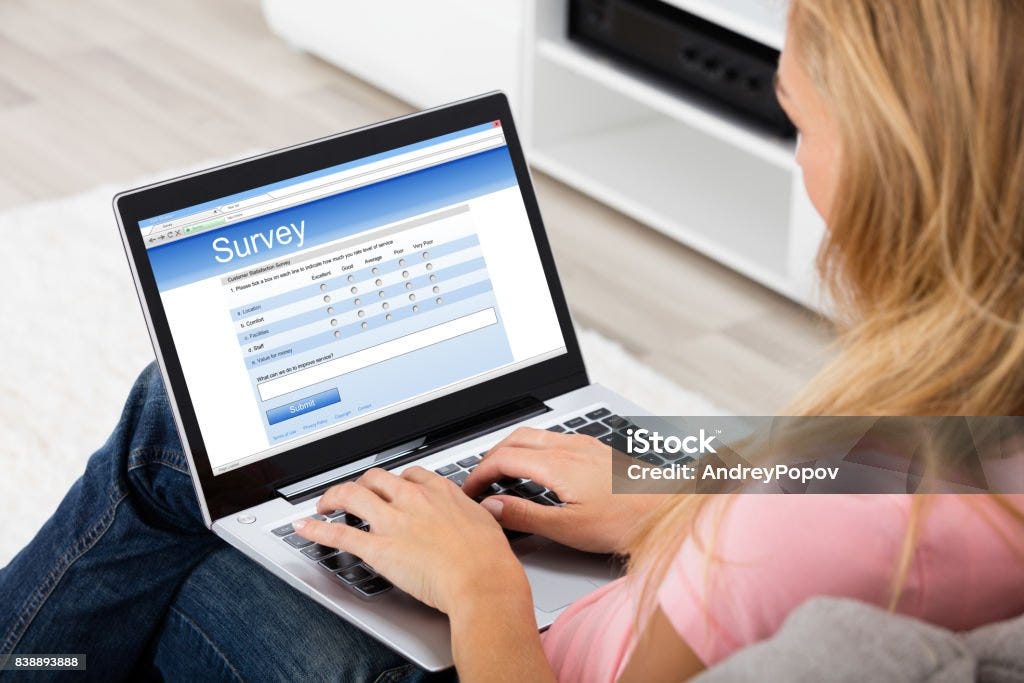
Next time you take a survey, try to avoid choosing “Other.”
Pick the response choice that seems closest to your real answer.
If you’re not sure how to force-fit your square peg of an answer into the round hole of the answer choices, ask the people giving the survey for help. You can ask them how to interpret the question. You can also describe what you actually want to say and ask how to choose which answer best fits.
What if after asking with the survey givers for help, you still can’t find a response choice that fits? Choosing “Other” is still better than skipping the question or abandoning the survey.
Whatever response you chose, consider using the write-in box to give the researchers feedback. They need it to realize the questions confused people and understand how to fix them.
…
What makes survey questions hard for you to answer?
What badly written survey questions have you seen?
How do you interpret “Other?”
Share your thoughts —and any other questions, comments, and experiences you like—by hitting the comment button below.